It is indisputable that speech recognition in AI (artificial intelligence) has come a long way since Bell Laboratories invented The Audrey, a device capable of recognizing a few spoken digits, in 1952.
Table of Contents
ToggleA recent study by Capgemini demonstrates how ubiquitous speech recognition has become. 74% of consumers sampled reported that they use conversational assistants to research and buy goods and services, create shopping lists, and check order status.
We are all familiar with digital assistants such as Google Assistant, Cortana, Siri, and Alexa. Google Assistant and Siri are used by over 1 billion people globally, and Siri has over 40 million users in the US alone. But, have you ever wondered how these tools understand what you say? Well, they use speech to text AI.
Understanding Speech Recognition
In speech recognition, the computer takes input in the form of sound vibrations. This is done by making use of an analog to digital converter that converts the sound waves into a digital format that the computer can understand.
A series of complex algorithms are then run on the data to recognize the speech and return a text result. Depending on the end-goal, the data may also be converted into another form. For example, Google Voice typing converts spoken words into text while personal assistants like Siri and Google Assistant take the sound input but can also give a voice response. Essentially, you issue a command such as “How is the weather?” and the device responds with an audible answer.
Advanced speech recognition in AI also comprises AI voice recognition where the computer can distinguish a particular speaker’s voice.
Uses of Speech Recognition
Speech recognition technology has been deployed in digital personal assistants, smart speakers, smart homes, and a wide range of products and solutions.
The technology has allowed us to perform a wide range of voice-activated tasks. You can now use your voice to cook a turkey, dim the lights, turn on the stereo, and do a host of other things in the home.
In aviation, Amazon holds a patent for a voice-controlled drone that can change its behavior mid-flight based on voice commands and gestures. Militaries around the world are also experimenting with speech to text in the cockpit. This is to ensure pilots spend more time focusing on the mission as opposed to fiddling with the instruments.
Also, as organizations produce more content, the need to make content available to audiences in many different formats has fueled the demand for speech to text and text to speech services.
In the medical field, doctors can now update patients’ medical records in real-time using voice notes while doing their rounds, instead of having to wait until they are back at the desk.
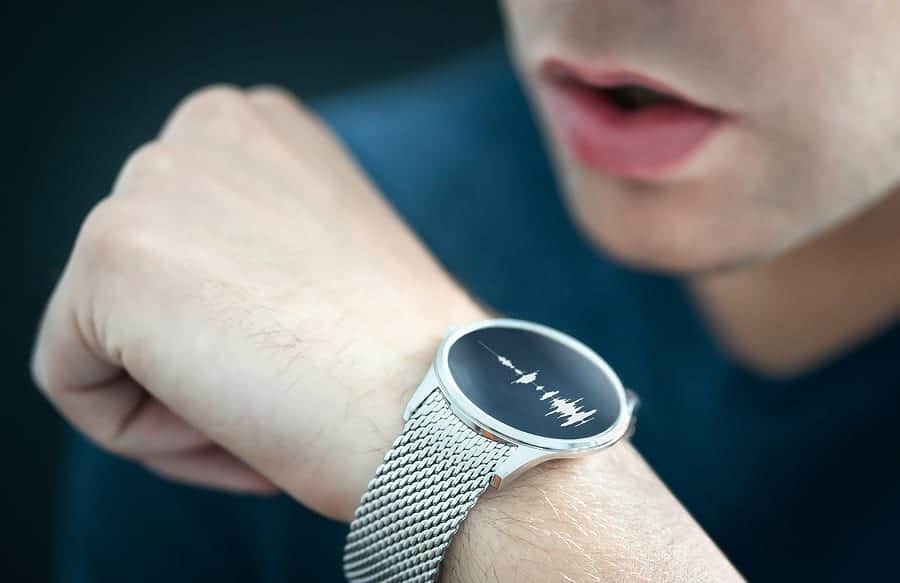
And in education, students with learning disabilities or those with poor writing skills can now learn on par with other students.
With such uptake, it isn’t surprising that the global speech recognition applications market is projected to grow to USD 3,505 million by 2024. Research by Gartner also shows that by 2022, 70% of white-collar workers will use conversational platforms daily.
The development of the Internet of Things (IoT) and big data is going to lead to deeper uptake of speech recognition technology.
Speech Recognition in Artificial Intelligence
The term “Artificial Intelligence” was first coined by John McCarthy (Dartmouth College), Claude Shannon (Bell Telephone Laboratories), Nathaniel Rochester (IBM), and Marvin Minsky (Harvard University) in a proposal to the Rockefeller Foundation in 1955. Artificial intelligence can be described as human intelligence shown by machines.
It was initially used to analyze and quickly compute data, but it is now used to perform tasks that previously could only be performed by humans.
Artificial intelligence is often confused with machine learning. Machine learning is a derivative of artificial intelligence and refers to the process of teaching a machine to recognize and learn from patterns rather than teaching it rules.
Computers are trained by feeding large volumes of data to an algorithm and then letting it pick out the patterns and learn. In the nascent days of machine learning, programmers had to write code for every object they wanted the computer to recognize – e.g., a cat vs. a human. These days, computers are shown numerous examples of each object. Over time, they learn without any human input.
Speech recognition, natural language processing, and translation use artificial intelligence today. Many speech recognition applications are powered by automatic speech recognition and Natural Language Processing (NLP). Automatic speech recognition refers to the conversion of audio to text, while NLP is processing the text to determine its meaning.
Humans rarely ever speak in a straightforward manner that computers can understand. Normal speech contains accents, colloquialisms, different cadences, emotions, and many other variations. It takes a great deal of natural language analysis to generate accurate text.
Challenges with Speech to Text AI
Despite the giant leap forward that AI speech to text has made over the last decade, there remain several challenges that stand in the way of true ubiquity.
The first of these is accuracy. The best applications currently boast a 95% accuracy rate – first achieved by Google Cloud Speech in 2017. Since then, many competitors have made great strides and achieved the same rate of accuracy.
While this is good progress, it means that there will always be a 5% error rate. This may seem like a small figure – and it is, where the issue at hand is a transcript that can be quickly edited by a human to correct errors. But, it is a big deal where voice is used to give a command to the computer. Imagine asking your car’s navigator to search the map for a particular location, and it searches for something different and sends you on your way in the wrong direction because it didn’t quite catch what you said. Or, imagine asking your smart home’s conversational assistant to turn off the lights, but it instead hears a different command and turns off the heating in winter.
Such errors are caused by background noise, heavy accents, unknown dialects, and varied voice pitch in different speakers. The next generation of speech recognition in AI needs to surmount these challenges and attain 100% accuracy in order to reach the last mile of uptake.
The other challenge is that humans don’t just listen to each other’s voices to understand what is being said. They also observe non-verbal communication to understand what is being communicated but isn’t being said. This includes facial expressions, gestures, and body language. So, while computers can hear and understand the content, we are a long way from getting to a point where they can pick up on non-verbal cues. The emotional robot that can hear, feel and interpret like a human is the holy grail of speech recognition.
Summary:
Speech recognition in AI
In speech recognition, the computer takes input in the form of sound vibrations. This is done by making use of an analog to digital converter that converts the sound waves into a digital format that the computer can understand. Advanced speech recognition in AI also comprises AI voice recognition where the computer can distinguish a particular speaker’s voice.