Learn why time-series predictive analytics enhances helps media companies stay relevant in a dynamic entertainment landscape.
Time series analytics has revolutionized stock market prediction and budgeting in organizations by forecasting future trends based on historical data. With advanced analytics, companies can make decisions in real-time to stay abreast of the changing landscape. However, predicting the events with artificial intelligence models is not as straightforward for the media and entertainment industries as it may be for other sectors due to several variables that influence content consumers.
Forecasting in the media and entertainment industry worsens when implementing time-series predictive analytics to enhance user engagement. This is primarily because the driving force for content virality changes rapidly over the years. However, time-series predictive analytics can provide a competitive advantage in the ever-changing media and entertainment industry if carried out with due diligence.
Predictive Analytics in Media And Entertainment Industry
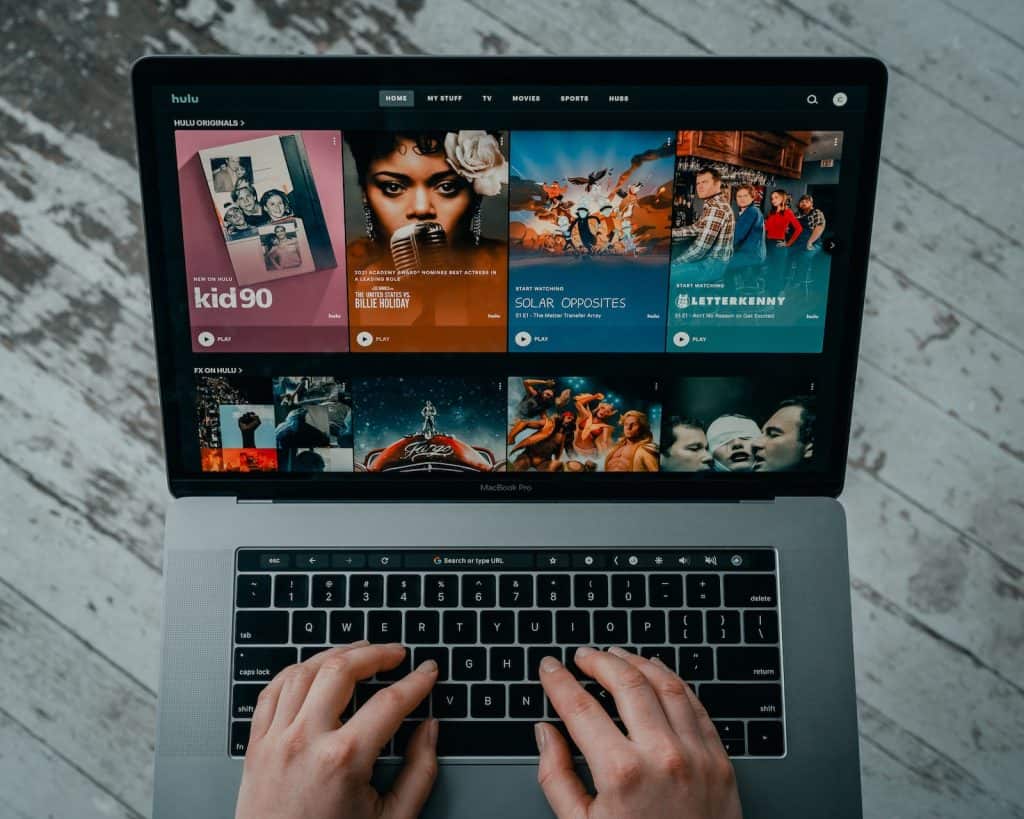
Both descriptive and predictive analytics are some of the most widely used aspects of any media and entertainment industry. While descriptive analytics is critical for gaining a holistic view of the business operations, predictive analytics allows content producers to evaluate if the company will accomplish its goals. However, time-series predictive analytics provides unique insights into the future that can assist companies in boosting their revenues with increased user engagement.
Although media and entertainment organizations are leveraging analytics for finding insights into users’ activities, time-series analytics enables companies to precisely predict the future as it accounts for seasonality. With descriptive analytics, you can find the reason behind a specific action but cannot identify how the same variable impacts in the future. In other words, time-series predictive analytics enhances the decision-making process in organizations. It also allows organizations to become future-proof to stay relevant in the dynamic media and entertainment landscape.
Time-series predictive analysis not only provides you with the ability to forecast with subscribed customers but also with unidentified users. The ability to understand how anonymous users behave in the future can allow media and entertainment organizations to enhance conversion rates to boost the business.
Techniques In Time Series Predictive Analytics
Time series analytics goes beyond traditional regression analysis due to the advantage of the temporal ordering of data. In the media and entertainment industries, organizations can collect data such as the time and the content consumed minutes to predict future engagement. This can be carried out for a single user or for the entire user base to forecast the traffic. Such time-series analytics allow companies to even understand how effectively their recommendation systems work. It can not only evaluate but also help recommendation engines to use time-series analytics for enhancing media and entertainment organizations’ machine learning initiatives for personalization.
Organizations are relying on machine learning to boost user engagement with content-based and collaborative recommendations. But, with the addition of time-series analytics, organizations can provide inputs to recommendations engines to further enhance the recommendations. In addition, it can allow companies to optimize marketing spend by predicting future traffic with time-series analytics.
Primarily there are three factors — autocorrelation, seasonality, and stationarity — in time-series analytics that help in obtaining desired results.
Autocorrelation: It is the similarity between various data points, which can help companies predict the same activity at a particular interval of time.
Seasonality: It is one of the most critical aspects, which is helpful in determining the changing patterns based on occasional events or events that happened at a particular time period.
Stationarity: It is a pattern of events where the mean and variance do not change over specific periods of time.
Some of the popular algorithms used with time-series analysis are:
- Autoregressive (AR)
- Moving Average (MA)
- Autoregressive Moving Average (ARMA)
- Autoregressive Integrated Moving Average (ARIMA)
- Exponential Smoothing (ES)
Autoregressive is a lagged prediction since the forecasting depends on the t-1 till t-n. The stochastic process of autoregressive models also brings in randomness to improve the accuracy of predictions. Used to predict behavioral patterns, autoregressive algorithms can assist media companies in finding the time when they could witness historical patterns again, keeping them prepared to manage traffic. It can also help companies make the most out of the same instance (rise in traffic) in the future with better offers or better recommendations.
Moving Average is similar to regression analysis but provides insights into the constant demand of certain content. Although not used to predict future trends, moving average offers a clear picture of the users’ demand, which the batch averaging does not. Moving average reduces the error rate due to averaging over a period of time.
Autoregressive Moving Averages is the combination of the above two to combine the prediction with moving averages for better accuracy, thereby making it a go-to algorithm for time-series forecasting. ARMA also accounts for assessing seasonality and stationarity.
Autoregressive Integrated Moving Average (ARIMA) is the most widely used algorithm for time series forecasting since it goes beyond seasonality and stationarity by autocorrelating events.
Exponential smoothing is used to decrease the weight of dated data by applying various transformations to have smoother trends. This reduces the risk of predicting the wrong trends due to changing user behaviors.
Outlook
Time series analysis is widely ignored in the media and entertainment industry but has a broader use case to predict and enhance engagement. However, the most pressing challenge for content producers will be to prepare datasets that could enable them to perform time-series analytics. The media and entertainment industry mostly rely on metadata and rich metadata for machine learning tasks but focusing on structured data for predictive modeling can make them more prepared and also perform perspective analytics to understand how specific insights, when incorporated, can help in changing the future event to boost business growth. Time series analytics is one of the rising trends in the top media and entertainment organizations to make new advancements and enhance decision making. With proper use of time series analytics, organizations can ensure operational resilience for overcoming potential changes in user behavior.